Cascades Towards Noise-Induced Transitions on Networks Revealed Using Information Flows
This paper examines noise-induced transitions in complex networks, identifying two key node roles: initiators, which propagate short-lived fluctuations to destabilize the system, and stabilizers, which encode long-term memory to restore stability. Using information-theoretic metrics, the study reveals a “domino effect” where noise cascades from low-degree to high-degree nodes, driving metastable transitions and tipping points. Our findings provide a framework for predicting and controlling critical transitions in systems like neural networks, ecosystems, and social dynamics.
WHEN NETWORKS FALL LIKE DOMINOES:
AN ESSENTIAL INTERACTION BETWEEN SHORT-LIVED AND LONG LIVED CORRELATIONS
— Cover of Journal Entropy featured our recent publication as a cover for the Special Issue 180th Anniversary of Ludwig Boltzmann
Authored by:
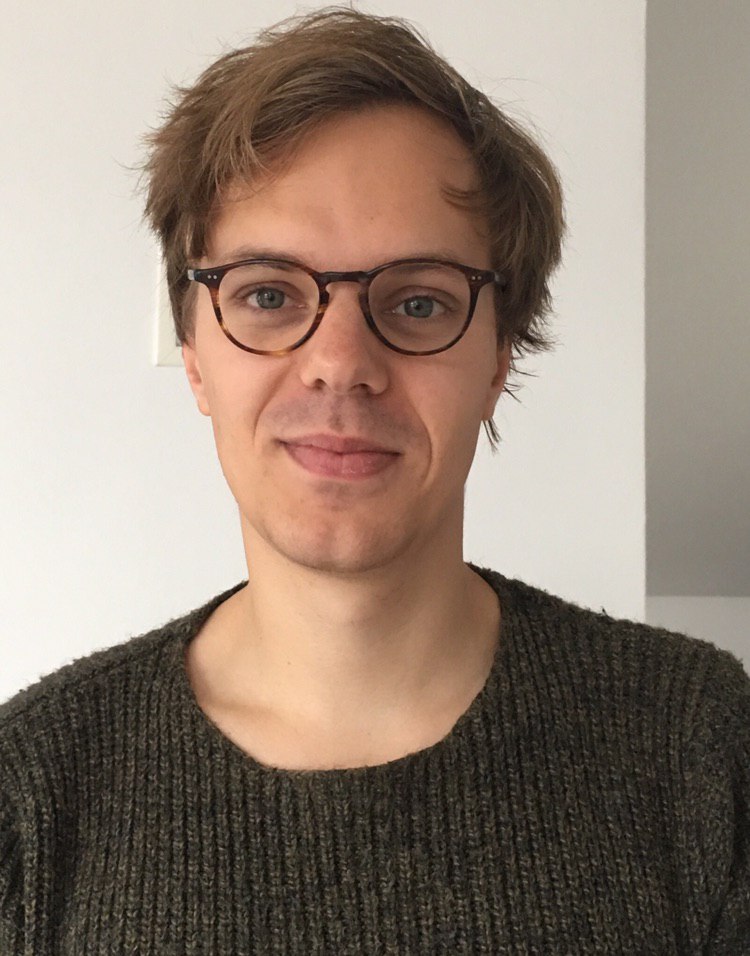
Casper van Elteren
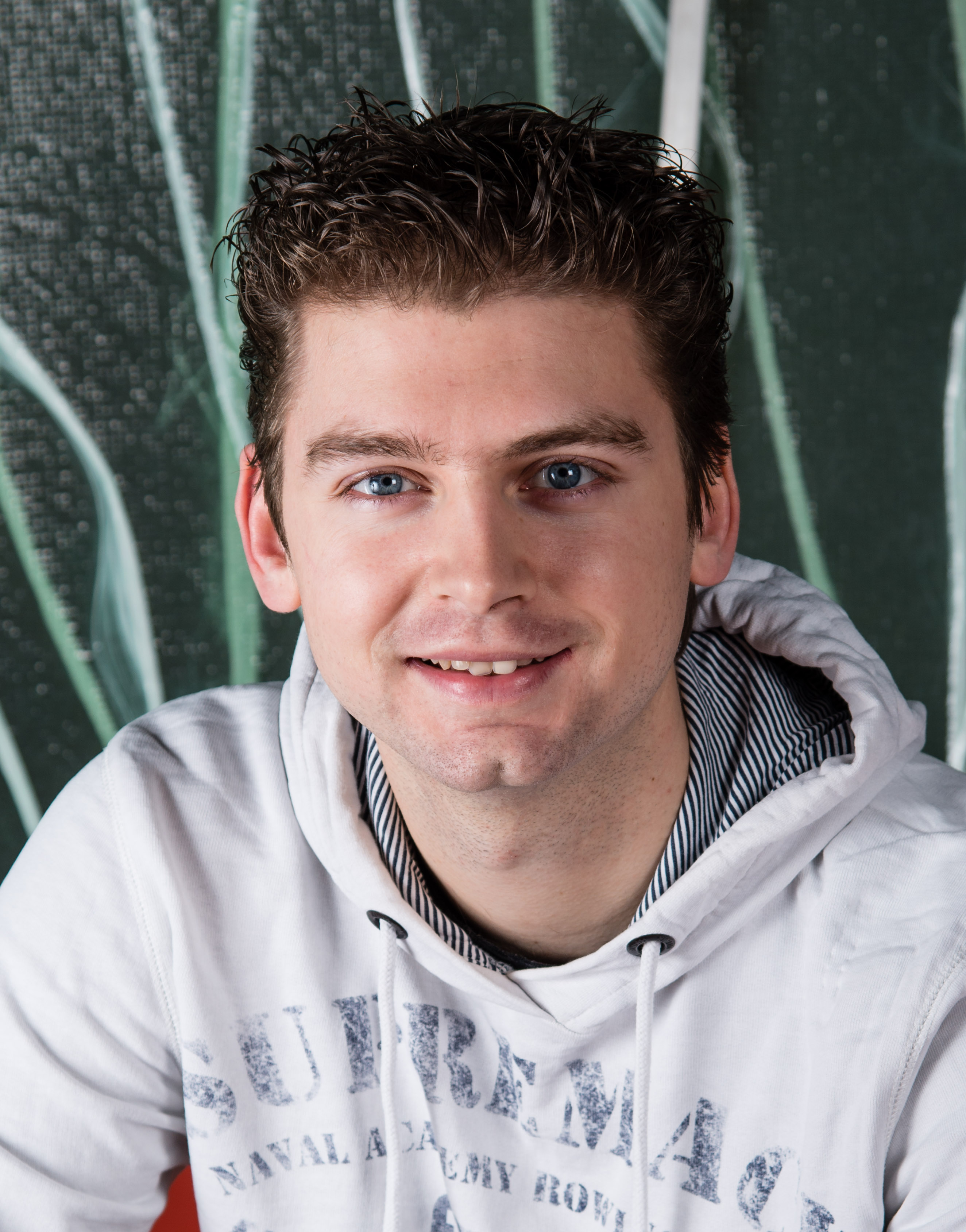
Rick Quax
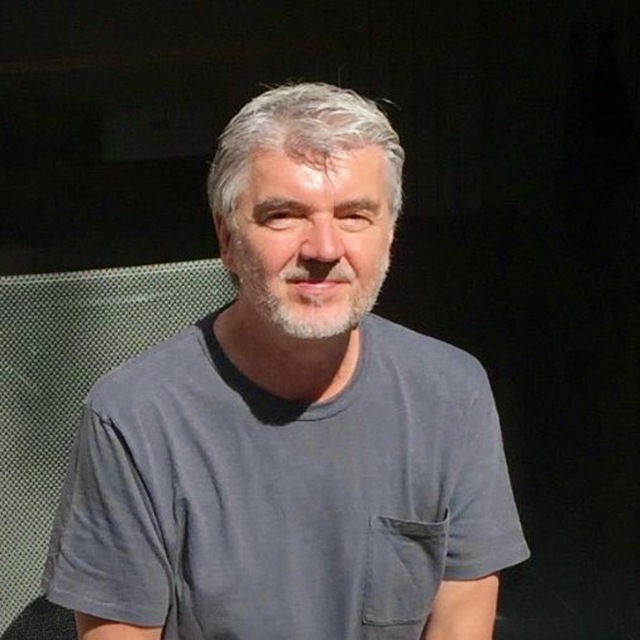
Peter Sloot
Research Highlights
Trajectories Towards Tipping Points and Their Predictive power
The cascade mechanism underpins metastable transitions, with low-degree nodes acting as initiators that trigger systemic change.
High-degree nodes provide stability by aligning with macrostates, while low-degree nodes drive transitions through noise propagation. Network structure plays a critical role in determining transition dynamics.
General Conclusions
1. Role Differentiation: The study identifies two critical node roles—initiators and stabilizers—within dynamical networks:
-
Initiators propagate short-lived fluctuations that destabilize the system and push it toward a tipping point.
-
Stabilizers encode long-term memory that reverses destabilization effects and stabilizes new attractor states.
2. Domino Effect: Metastable transitions occur through a domino-like mechanism where noise propagates from low-degree to high-degree nodes. This process is driven by integrated mutual information (short-term dynamics) and asymptotic information (long-term stability).
3. Predictive Metrics: A rise in asymptotic information serves as an early warning signal for tipping points, enabling predictions about critical transitions.